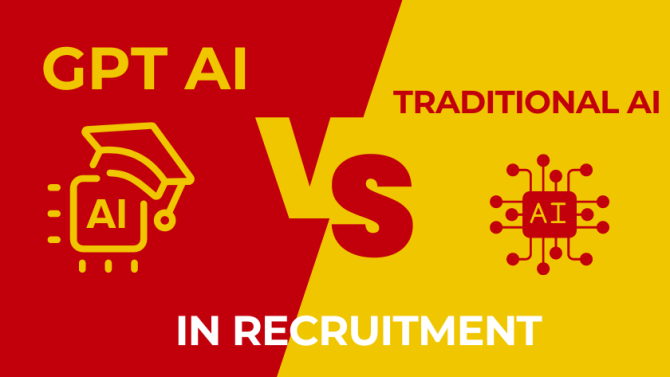
In the evolving landscape of human resource technology, advanced AI models like GPT (Generative Pre-trained Transformers) have revolutionized numerous processes, including talent acquisition. There are vital differences between GPT-based and traditional methods, which we will consider exemplifying AI resume screening, highlighting the advancements and efficiencies brought about by the newer technology.
1. Understanding the Technologies
Traditional AI Resume Screening: Traditional AI screening tools primarily rely on keyword matching and rule-based systems. They scan resumes for specific terms and phrases that match the job description or criteria predetermined by the employer. While effective in filtering a large volume of applications, these systems can miss nuances and often overlook candidates who might be a good fit but don't precisely match the keyword criteria.
GPT-based Resume Screening: GPT models, like the latest iterations (e.g., GPT-4), leverage deep learning and natural language processing (NLP) to understand and interpret text more contextually and holistically. Instead of merely matching keywords, they analyze the entire content of a resume, understanding the context, extracting meaningful insights, and even assessing the candidate's tone and writing style.
2. Contextual Understanding and Nuance
Traditional AI: Lacks deep contextual understanding. It may overlook candidates who use terminology different from what the system is programmed to recognize.
GPT Models: Excel in understanding context and nuance. They can infer qualifications, skills and experiences even when not explicitly stated, offering a more rounded view of each candidate.
3. Customization and Flexibility
Traditional AI: Often requires manual setting of parameters and keywords for each job opening, which can be time-consuming and less adaptable to different job types.
GPT Models: Offer greater flexibility. They can adapt to different job descriptions and requirements more fluidly, requiring less manual intervention and offering more tailored screening. For example, you can easily distinguish candidates with experience in a particular industry, education field and career plans.
4. Candidate Experience
Traditional AI: May result in a more impersonal candidate experience, as feedback or communication is often generic and automated.
GPT Models: Have the potential to personalize communication with candidates, providing more detailed feedback and creating a more engaging experience.
5. Bias and Fairness
Traditional AI: Can inadvertently reinforce biases if the keyword selection or criteria are biased. This is a significant concern in traditional screening methods.
GPT Models: While not immune to biases (as they learn from large datasets that may contain biases), GPT models can be designed and trained to minimize bias, offering a fairer evaluation process.
6. Continuous Learning and Improvement
Traditional AI: Updates and improvements in traditional systems are usually manual and less frequent.
GPT Models: Benefit from continuous learning capabilities. As they process more data, they can refine their understanding and screening abilities, staying current with language and industry trends.
Conclusion
The transition from traditional AI resume screening to GPT-based models marks a significant leap in how organizations can handle recruitment. While traditional methods have their merits in handling large volumes of data, GPT models bring a level of sophistication, understanding, and flexibility that is unparalleled. This shift not only enhances the efficiency and effectiveness of the screening process but also promises a more equitable and candidate-friendly approach to recruitment.